Business Problems:
Full report here and SQL code here
The key business problems identified for Cyclistic are as follows:
- Low Ridership and Usage Patterns: There is a need to understand the usage patterns of both casual users and members, identify factors affecting ridership, and find ways to increase overall ridership.
- Seasonal Variations: There are variations in bike usage between different seasons, with warmer months showing higher usage. Strategies are required to address this seasonal fluctuation and maximize bike utilization throughout the year.
- Targeting Casual Users: Casual users represent an opportunity for increased ridership. Understanding their preferences, needs, and barriers can help design effective strategies to convert them into members.
Analytical Approach:
To address these business problems, the following analytical approach was undertaken:
- Data Analysis and Exploration: Comprehensive data on bike usage, user types, and timeframes was analyzed to identify patterns, trends, and correlations.
- User Segmentation: Users were segmented into casual users and members based on their usage patterns and characteristics.
- Seasonal Analysis: Usage patterns were analyzed across different seasons to understand the impact of weather on bike usage and identify strategies for maximizing ridership throughout the year.
- Usage Patterns and Preferences: Analysis was conducted to identify preferences and usage patterns of both casual users and members, including popular days, trip durations, and preferred bike stations.
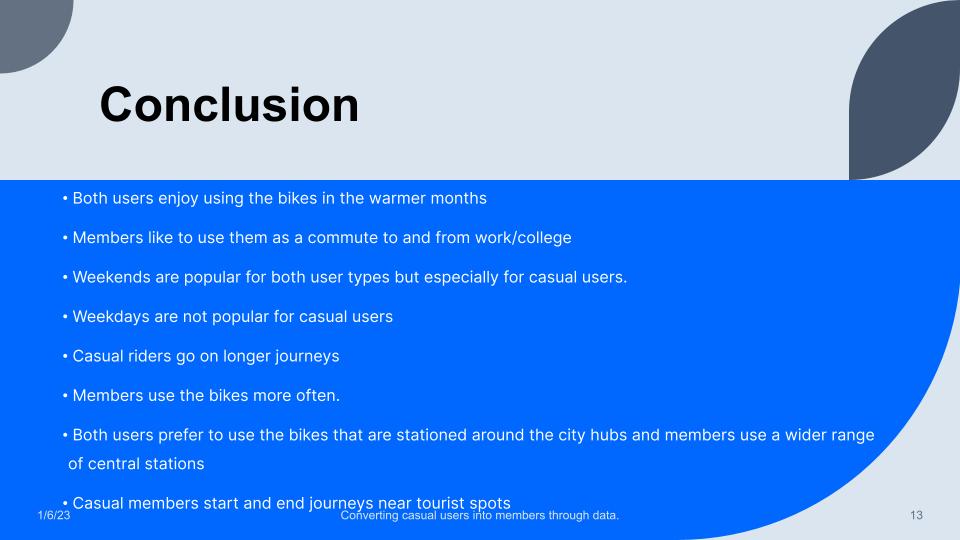
Based on the analysis, the following key insights were derived:
- Seasonal Usage Patterns: Both casual users and members prefer using bikes in warmer months, indicating the need to focus on strategies to sustain ridership during colder seasons.
- Commute Usage: Members primarily use bikes for commuting to and from work or college, suggesting opportunities to enhance the service for their specific needs.
- Weekend Usage: Weekends are popular for both user types, with a higher emphasis on casual users. Targeted initiatives can be implemented to further encourage weekend usage.
- Station Preferences: Both casual users and members prefer bike stations located around city hubs, while members tend to utilize a wider range of central stations.
Recommendations:
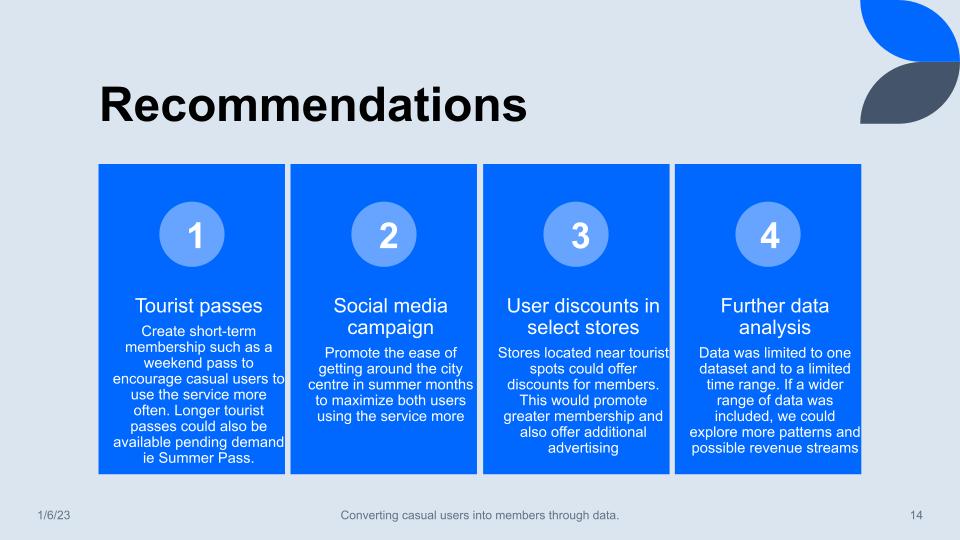
Based on the insights gained, the following recommendations are suggested:
- Tourist Passes: Introduce short-term membership options such as weekend passes or seasonal tourist passes to attract casual users and encourage more frequent usage.
- Social Media Campaign: Launch a targeted social media campaign to promote the convenience and benefits of using the bike-sharing service, particularly during the warmer months and for city centre transportation.
- User Discounts: Collaborate with select stores near tourist spots to offer discounts exclusively for members, creating incentives for casual users to become members and driving additional revenue.
- Further Data Analysis: Expand the scope of data analysis by incorporating additional datasets and longer time ranges to explore more comprehensive usage patterns and identify new revenue streams.
By implementing these recommendations, Cyclistic can aim to convert casual users into members, increase overall ridership, and optimize the service based on user preferences and seasonal variations.