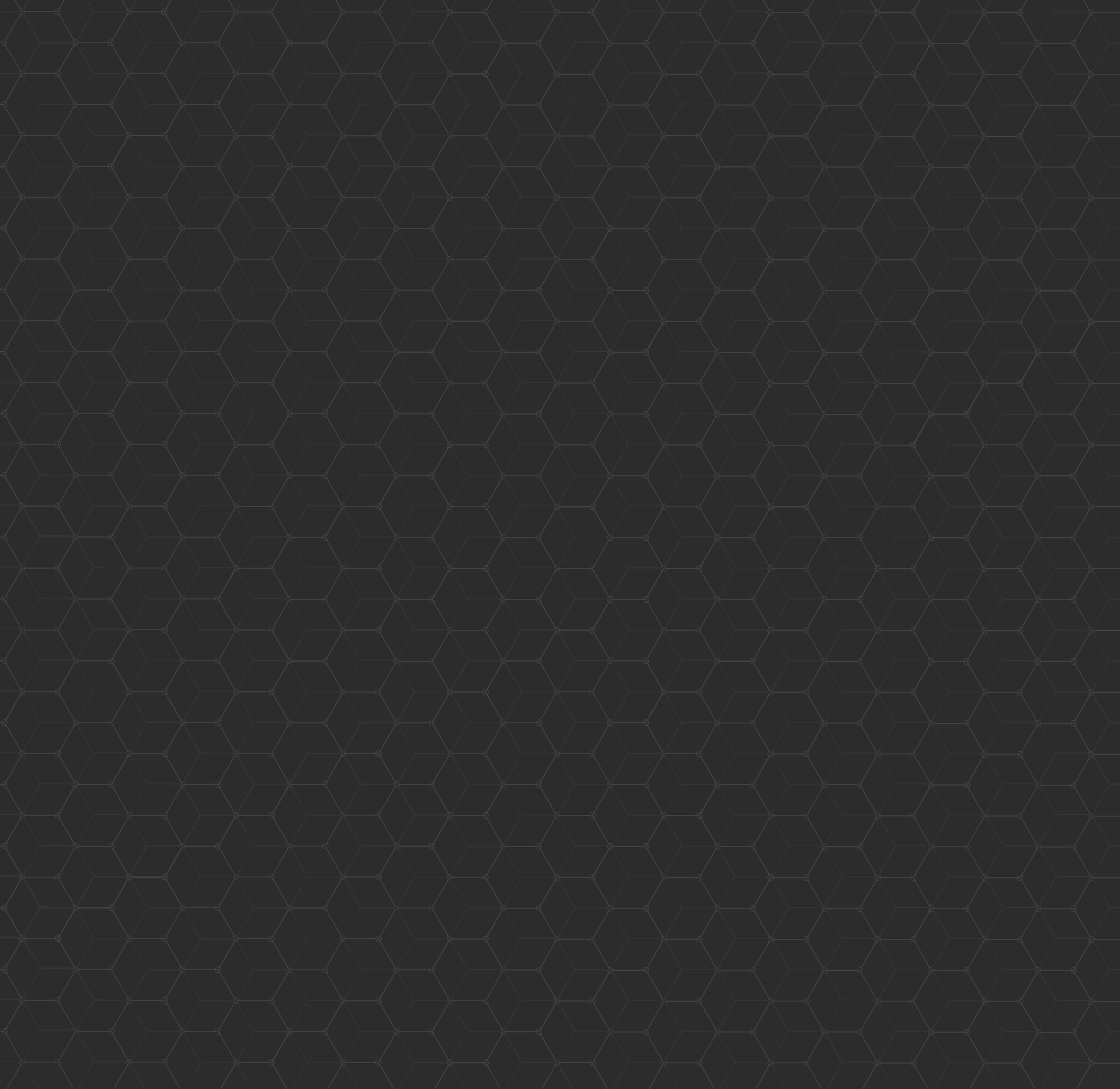
Self-Paced Course
Data Science in Python: Unsupervised Learning
Master the foundations of unsupervised learning in Python, including clustering, anomaly detection, dimensionality reduction, and recommenders
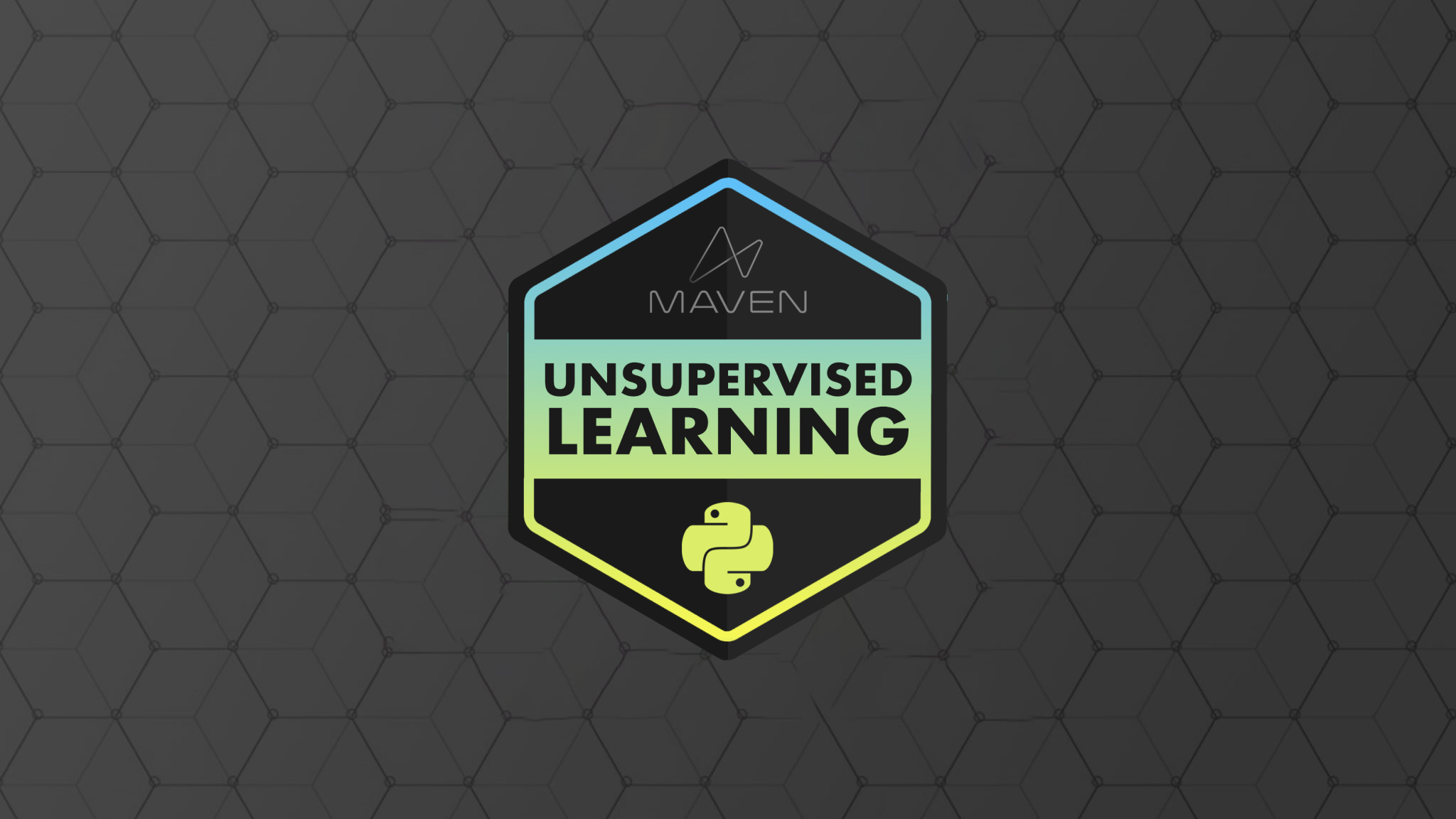
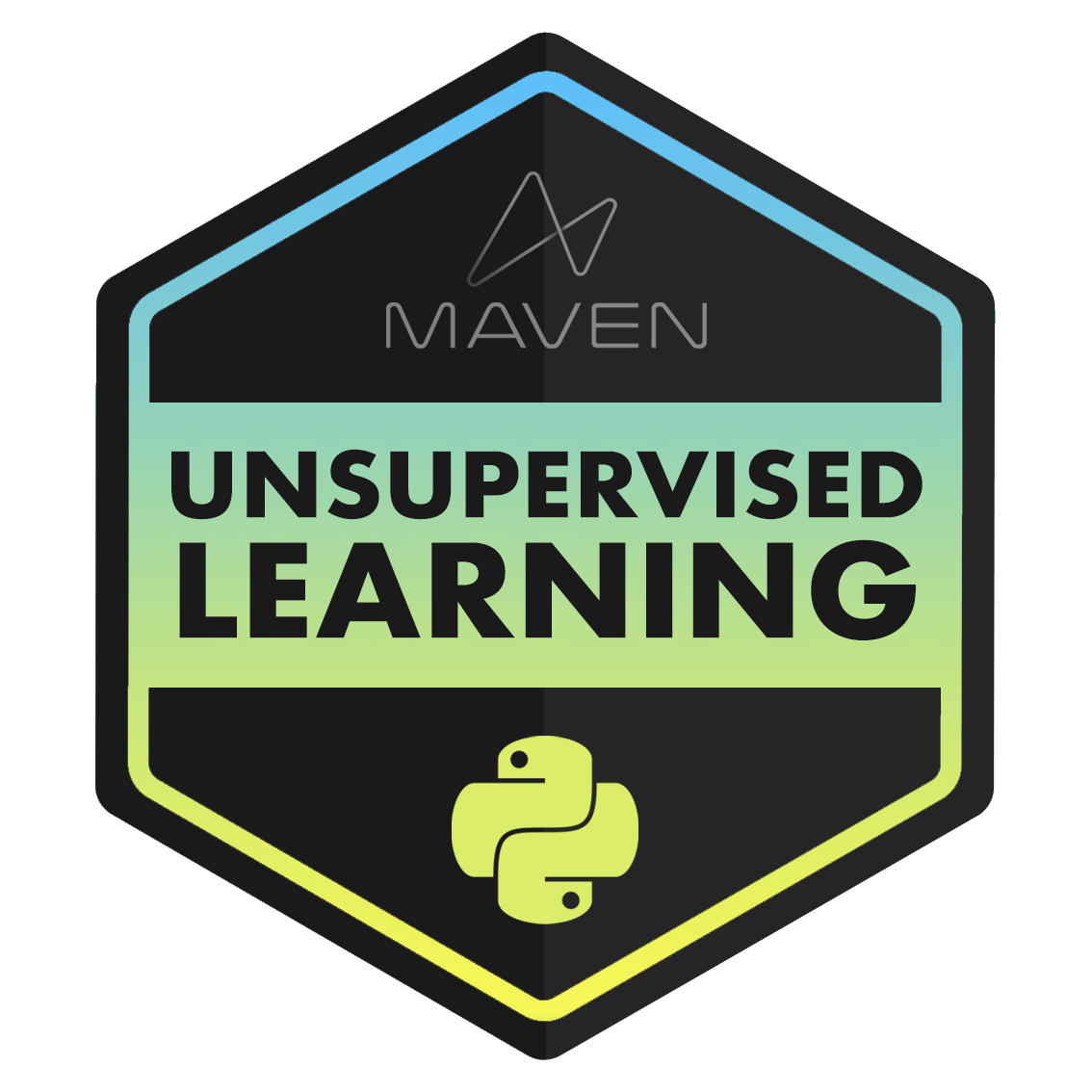
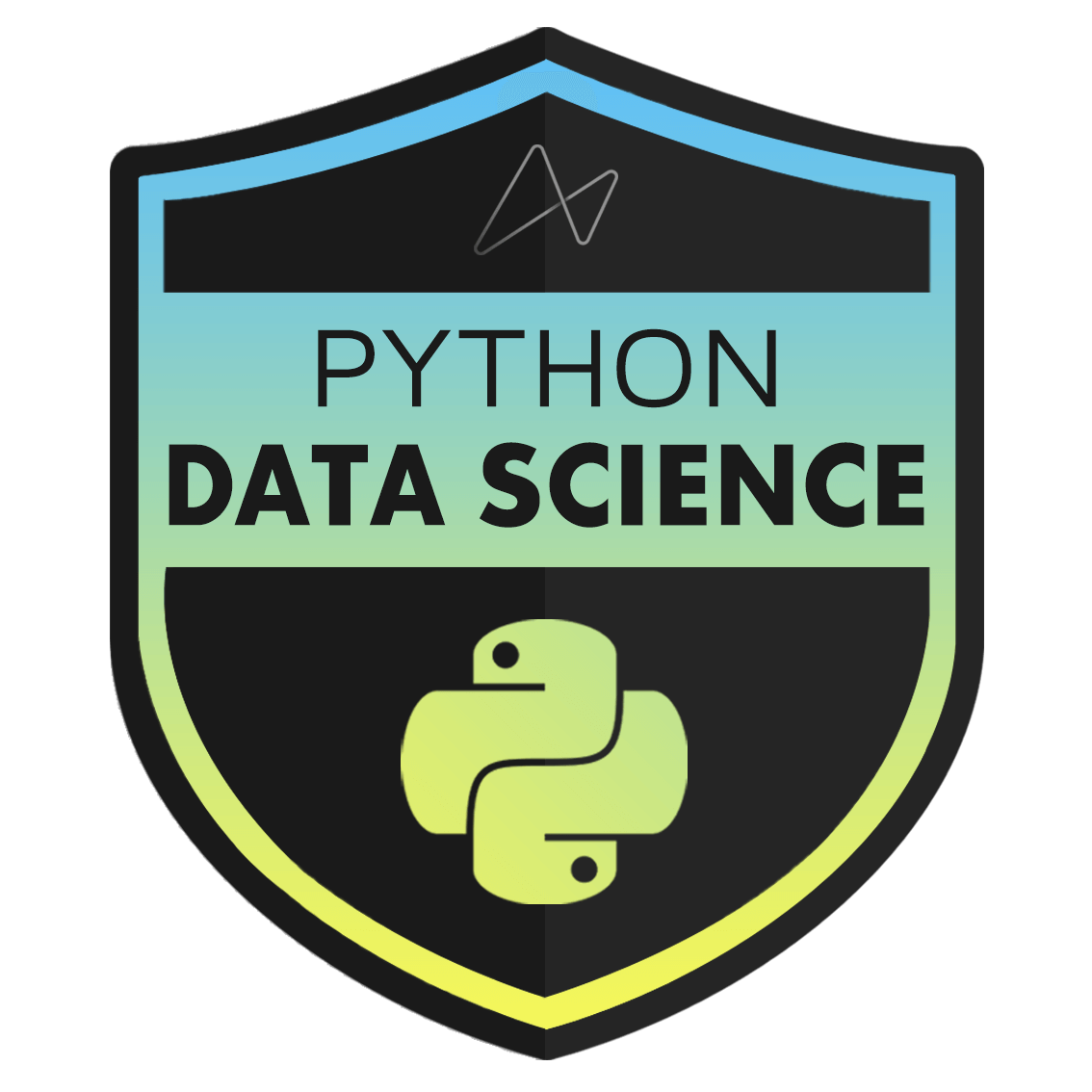
Course Description
This is a hands-on, project-based course designed to help you master the foundations for unsupervised learning in Python.
We’ll start by reviewing the data science workflow, discussing the techniques & applications of unsupervised learning, and walking through the data prep steps required for modeling. You’ll learn how to set the correct row granularity for modeling, apply feature engineering techniques, select relevant features, and scale your data using normalization and standardization.
From there we'll fit, tune, and interpret 3 popular clustering models using scikit-learn. We’ll start with K-Means Clustering, learn to interpret the output’s cluster centers, and use inertia plots to select the right number of clusters. Next, we’ll cover Hierarchical Clustering, where we’ll use dendrograms to identify clusters and cluster maps to interpret them. Finally, we’ll use DBSCAN to detect clusters and noise points and evaluate the models using their silhouette score.
We’ll also use DBSCAN and Isolation Forests for anomaly detection, a common application of unsupervised learning models for identifying outliers and anomalous patterns. You’ll learn to tune and interpret the results of each model and visualize the anomalies using pair plots.
Next, we’ll introduce the concept of dimensionality reduction, discuss its benefits for data science, and explore the stages in the data science workflow in which it can be applied. We’ll then cover two popular techniques: Principal Component Analysis, which is great for both feature extraction and data visualization, and t-SNE, which is ideal for data visualization.
Last but not least, we’ll introduce recommendation engines, and you'll practice creating both content-based and collaborative filtering recommenders using techniques such as Cosine Similarity and Singular Value Decomposition.
Throughout the course you'll play the role of an Associate Data Scientist for the HR Analytics team at a software company trying to increase employee retention. Using the skills you learn throughout the course, you'll use Python to segment the employees, visualize the clusters, and recommend next steps to increase retention.
If you're an aspiring or seasoned data scientist looking for a practical overview of unsupervised learning techniques in Python with a focus on interpretation, this is the course for you.
COURSE CONTENTS:
16.5 hours on-demand video
22 homework assignments
7 quizzes
3 projects
2 skills assessments (1 benchmark, 1 final)
COURSE CURRICULUM:
- Welcome to the Course!
- Benchmark Assessment
- Course Introduction
- About This Series
- Course Structure & Outline
- DOWNLOAD: Course Resources
- Introducing the Course Project
- Setting Expectations
- Jupyter Installation & Launch
- Section Introduction
- What is Data Science?
- Data Science Skill Set
- What is Machine Learning?
- Common Machine Learning Algorithms
- Data Science Workflow
- Step 1: Scoping a Project
- Step 2: Gathering Data
- Step 3: Cleaning Data
- Step 4: Exploring Data
- Step 5: Modeling Data
- Step 6: Sharing Insights
- Unsupervised Learning
- Key Takeaways
- Section Introduction
- Unsupervised Learning 101
- Unsupervised Learning Techniques
- Unsupervised Learning Applications
- Structure of This Course
- Unsupervised Learning Workflow
- Key Takeaways
- Section Introduction
- Data Prep for Unsupervised Learning
- Setting the Correct Row Granularity
- DEMO: Group By
- DEMO: Pivot
- ASSIGNMENT: Setting the Correct Row Granularity
- SOLUTION: Setting the Correct Row Granularity
- Preparing Columns for Modeling
- Identifying Missing Data
- Handling Missing Data
- Converting to Numeric
- Converting to DateTime
- Extracting DateTime
- Calculating Based on a Condition
- Dummy Variables
- ASSIGNMENT: Preparing Columns for Modeling
- SOLUTION: Preparing Columns for Modeling
- Feature Engineering
- Feature Engineering During Data Prep
- Applying Calculations
- Binning Values
- Identifying Proxy Variables
- Feature Engineering Tips
- ASSIGNMENT: Feature Engineering
- SOLUTION: Feature Engineering
- Excluding Identifiers From Modeling
- Feature Selection
- ASSIGNMENT: Feature Selection
- SOLUTION: Feature Selection
- Feature Scaling
- Normalization
- Standardization
- ASSIGNMENT: Feature Scaling
- SOLUTION: Feature Scaling
- Key Takeaways
- Section Introduction
- Clustering Basics
- K-Means Clustering
- K-Means Clustering in Python
- DEMO: K-Means Clustering in Python
- Visualizing K-Means Clustering
- Interpreting K-Means Clustering
- Visualizing Cluster Centers
- ASSIGNMENT: K-Means Clustering
- SOLUTION: K-Means Clustering
- Inertia
- Plotting Inertia in Python
- DEMO: Plotting Inertia in Python
- ASSIGNMENT: Inertia Plot
- SOLUTION: Inertia Plot
- Tuning a K-Means Model
- DEMO: Tuning a K-Means Model
- ASSIGNMENT: Tuning a K-Means Model
- SOLUTION: Tuning a K-Means Model
- Selecting the Best Model
- DEMO: Selecting the Best Model
- ASSIGNMENT: Selecting the Best K-Means Model
- SOLUTION: Selecting the Best K-Means Model
- Hierarchical Clustering
- Dendrograms in Python
- Agglomerative Clustering in Python
- DEMO: Agglomerative Clustering in Python
- Cluster Maps in Python
- DEMO: Cluster Maps in Python
- ASSIGNMENT: Hierarchical Clustering
- SOLUTION: Hierarchical Clustering
- DBSCAN
- DBSCAN in Python
- Silhouette Score
- Silhouette Score in Python
- DEMO: DBSCAN and Silhouette Score in Python
- ASSIGNMENT: DBSCAN
- SOLUTION: DBSCAN
- Comparing Clustering Algorithms
- Clustering Next Steps
- DEMO: Compare Clustering Models
- DEMO: Label Unseen Data
- Key Takeaways
- Project Overview
- SOLUTION: Data Prep
- SOLUTION: K-Means Clustering
- SOLUTION: Hierarchical Clustering
- SOLUTION: DBSCAN
- SOLUTION: Compare, Recommend and Predict
- Section Introduction
- Anomaly Detection Basics
- Anomaly Detection Approaches
- Anomaly Detection Workflow
- Isolation Forests
- Isolation Forests in Python
- Visualizing Anomalies
- Tuning and Interpreting Isolation Forests
- ASSIGNMENT: Isolation Forests
- SOLUTION: Isolation Forests
- DBSCAN for Anomaly Detection
- DBSCAN for Anomaly Detection in Python
- Visualizing DBSCAN Anomalies
- ASSIGNMENT: DBSCAN for Anomaly Detection
- SOLUTION: DBSCAN for Anomaly Detection
- Comparing Anomaly Detection Algorithms
- RECAP: Clustering and Anomaly Detection
- Key Takeaways
- Section Introduction
- Dimensionality Reduction Basics
- Why Reduce Dimensions?
- Dimensionality Reduction Workflow
- Principal Component Analysis
- Principal Component Analysis in Python
- Explained Variance Ratio
- DEMO: PCA and Explained Variance Ratio in Python
- ASSIGNMENT: Principal Component Analysis
- SOLUTION: Principal Component Analysis
- Interpreting PCA
- DEMO: Interpreting PCA
- ASSIGNMENT: Interpreting PCA
- SOLUTION: Interpreting PCA
- Feature Selection vs Feature Extraction
- PCA Next Steps
- T-SNE
- T-SNE in Python
- ASSIGNMENT: T-SNE
- SOLUTION: T-SNE
- PCA vs t-SNE
- DEMO: Dimensionality Reduction and Clustering
- ASSIGNMENT: T-SNE & K-Means Clustering
- SOLUTION: T-SNE & K-Means Clustering
- Key Takeaways
- Section Introduction
- Recommenders Basics
- Content-Based Filtering
- Cosine Similarity
- Cosine Similarity in Python
- Making a Content-Based Filtering Recommendation
- ASSIGNMENT: Content-Based Filtering
- SOLUTION: Content-Based Filtering
- Collaborative Filtering
- User-Item Matrix
- ASSIGNMENT: User-Item Matrix
- SOLUTION: User-Item Matrix
- Singular Value Decomposition
- Singular Value Decomposition in Python
- ASSIGNMENT: Singular Value Decomposition
- SOLUTION: Singular Value Decomposition
- Choosing the Number of Components
- DEMO: Choosing the Number of Components
- ASSIGNMENT: Choosing the Number of Components
- SOLUTION: Choosing the Number of Components
- Making a Collaborative Filtering Recommendation
- DEMO: Making a Collaborative Filtering Recommendation
- ASSIGNMENT: Collaborative Filtering
- SOLUTION: Collaborative Filtering
- Recommender Next Steps
- DEMO: Hybrid Approach
- Key Takeaways
- Project Overview
- SOLUTION: Data Prep
- SOLUTION: Apply TuncatedSVD
- SOLUTION: Visualize the Results
- SOLUTION: Make Recommendations
- Section Introduction
- Unsupervised Learning Flow Chart
- Unsupervised Learning Techniques & Applications
- Unsupervised Learning in the Data Science Workflow
- Key Takeaways
- Final Project Overview
- SOLUTION: Data Prep
- SOLUTION: Clustering
- SOLUTION: Visualization
- SOLUTION: Exploration
- SOLUTION: Recommendations
- Final Assessment
- Course Feedback Survey
- Share the Love!
- Next Steps
WHO SHOULD TAKE THIS COURSE?
Data scientists who want to learn how to build and interpret unsupervised learning models in Python
Analysts or BI experts looking to learn about unsupervised learning or transition into a data science role
Anyone interested in learning one of the most popular open source programming languages in the world
WHAT ARE THE COURSE REQUIREMENTS?
- We strongly recommend taking our Data Prep & EDA course first
- Jupyter Notebooks (free download, we'll walk through the install)
- Familiarity with base Python and Pandas is recommended, but not required
Start learning for FREE, no credit card required!
Every subscription includes access to the following course materials
- Interactive Project files
- Downloadable e-books
- Graded quizzes and assessments
- 1-on-1 Expert support
- 100% satisfaction guarantee
- Verified credentials & accredited badges
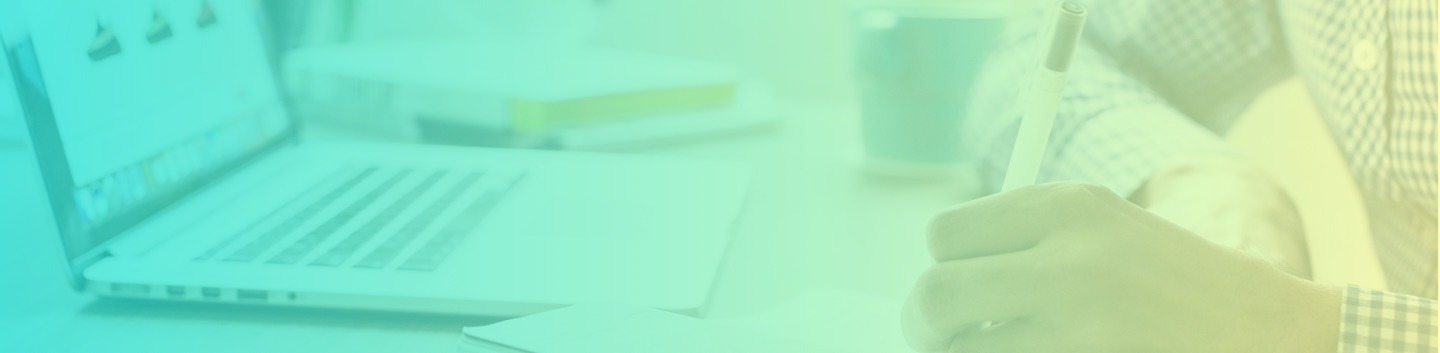
Ready to become a
data rockstar?
Start learning for free, no credit card required!